To answer that question, this Article develops a brand new, complete account of the higher-order ideas animating Wal-Mart and its progeny. Instead, the case
law firm immigration is driven by a demand for accuracy within the decision of claims—not solely within the mixture, however as to individual class members. At the same time, it rejected novel approaches that might not adequately minimize individual error. Thus, well-designed algorithms can ship accuracy to at least the degree that the post-Wal-Mart Court has found sufficient—and likely do even better. After Wal-Mart, the Supreme Court continued to endorse a small handful of long-established statistical designs that it believed might produce such particular person accuracy. But as this Article has shown, Wal-Mart and its progeny do not flatly forbid such new, bold approaches. But careful analysis reveals that the Court did not flatly forbid ambitious, new statistical strategies for effectively resolving individual questions. Modern machine learning merely is a set of associated algorithmic strategies for producing automated individual choices with excessive accuracy. Instead, they demand only that new methods of statistical proof are correct at the level of individual class members. Thus, machine learning represents a path to class certification across a wide swath of important, but currently uncertifiable, classes of claims. Advanced machine learning algorithms can determine, with high accuracy, whether individual class members can set up essential parts of legal responsibility and, if so, what their damages should be. Using it, numerous individual litigants with valid, however at present unvindicable,
law firm immigration claims might access the court docket system and, in the end, safe the justice they are presently denied. As already noted, the Blue J algorithm can precisely apply settled legal exams to advanced factual situations. Why should AI class actions survive scrutiny underneath Wal-Mart when other methods of statistical proof have failed? Common particular person questions in school actions—medical causation, reliance, and so forth.—work the same method, suggesting that related quantities of coaching data could be required. By most accounts, Wal-Mart threw a critical roadblock within the path of those in search of to attain class certification in historically hard-to-certify cases by using artistic statistical proof. There are good reasons to suppose that, in AI class actions, cheap amounts of information would suffice.
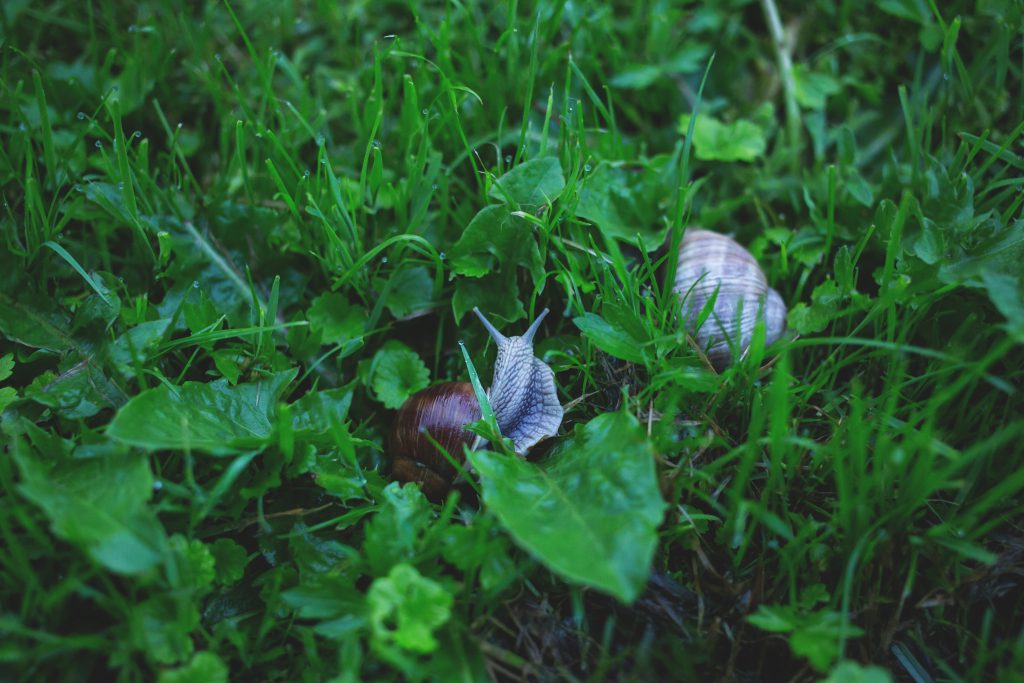
This is well within the vary of the possible, so far as pattern adjudications go. Blue J was trained utilizing the complete corpus of related Tax Court of Canada decisions—numbering 600. This Article proposes a first-of-its-kind technique of statistical proof designed to overcome Wal-Mart’s critique. Such artificially clever class actions would revolutionize combination litigation. Recall that pre-Wal-Mart courts accredited as practicable as many as 1,a hundred and fifty hearing-based sample adjudications of particular person questions. First, the sorts of particular person questions to be resolved in a category motion aren't unprecedented on the earth of machine studying. By enabling class certification across a broad range of doctrinal areas, they might facilitate the vindication of numerous meritorious—but at present unvindicable—claims. In doing so, AI class actions would serve the interests of plaintiffs,
law firm work permit defendants, and society at massive. This straightforwardly illustrates how machine learning would facilitate class certification where it is currently thought-about unimaginable. Assume for now that these algorithmic choices would, like a jury determination, be the final word on every particular person concern. In quick, cutting-edge machine learning algorithms would effectively resolve individual questions at school actions, thereby allowing common ones to predominate. Like Blue J, a trained algorithm in an AI class action would effectively resolve particular person questions as to each individual class member. Only a small pattern of sophistication members’ individual questions would be tried to the jury. Other disputes would likely be resolved by way of "battles of the specialists." In such battles, each parties submit their own professional opinions about correct algorithmic design. At the identical time, they would help avoid restoration
law firm turkey citizenship by investment plaintiffs who were not owed it.
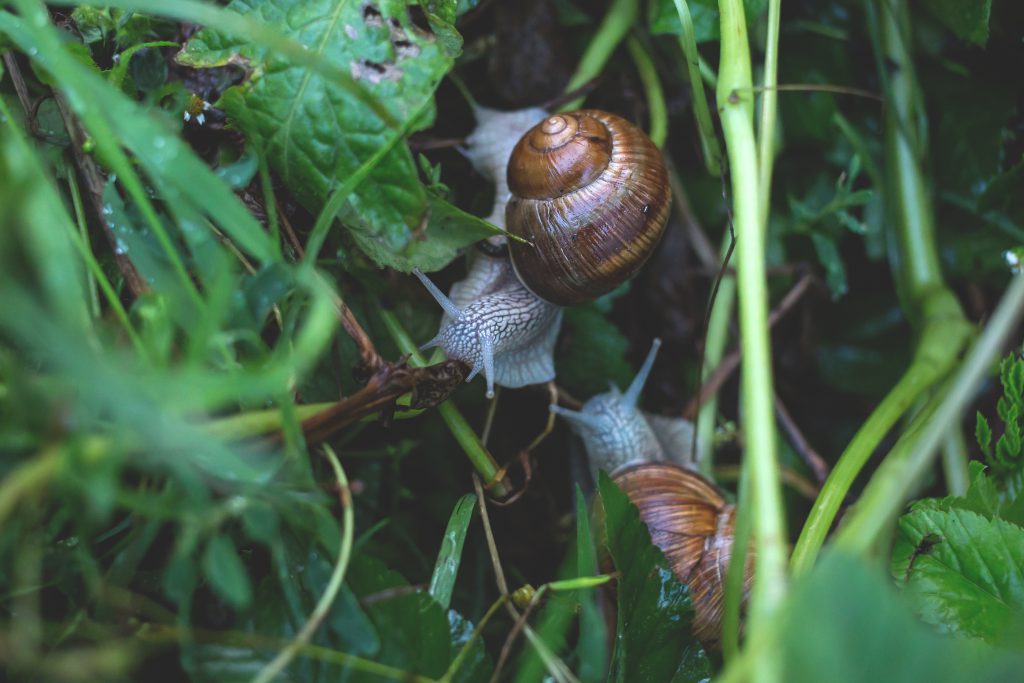
With individual questions thus minimized at trial, district courts might rule confidently that frequent questions predominated, satisfying Rule 23. Indeed, in advanced litigation—including class actions—they are frequently fought particularly about model design. The competing opinions are evaluated by some combination of the court—applying the Daubert test—and the factfinder—choosing the best argument that survives Daubert. Everyone else’s questions would be completely resolved by a pc in essentially no time in any respect. Issues—like medical causation, reliance, or intent—which presently require particular person adjudications for every class member would as an alternative be resolved with excessive effectivity. Older-fashioned statistical models—like traditional regressions—are mainstays of securities, antitrust, and disparate impact litigation. These models, like superior machine studying algorithms, can only be as correct because the underlying knowledge. Likewise, as with coaching a machine studying algorithm, traditional fashions depend on their designers’ selections about how best to make the model fit the data. Different regression methods, for example, fit the info to several types of curves. They due to this fact additionally elevate alternatives for
lawyer turkiye citizenship by investment skilled disagreement about whether or not defective information has been adequately corrected.